Revant Nayar, CTO of FMI Tech and CIO of Princeton...
Read MoreQuantum Revolution in
Asset Management
FMI Technologies
Quantum Revolution in Asset Management
Asset Management
At FMI Technologies we manage portfolios by leveraging the cutting-edge quantum AI and the principles of physics to identify lasting alpha opportunities in the market. While pursuing alpha generation, we stay committed to rigorous risk control and market neutrality.
We work with Family Offices, Institutions, Hedge Funds, and Private Individuals.
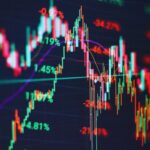
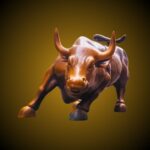
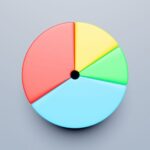
Quantum AI & Algorithms
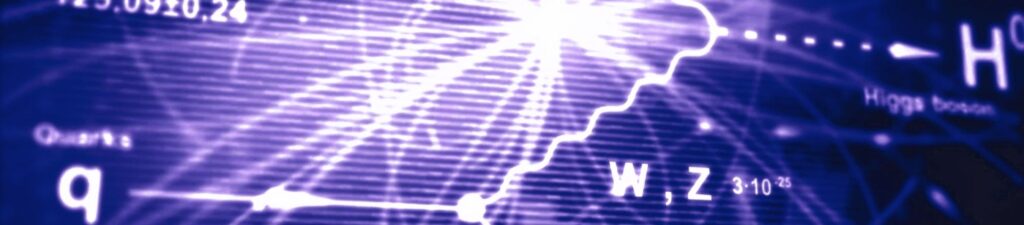
FMI Tech is engaged in innovative research that revolutionizes the way we think about financial markets. This research brings out applications of quantum AI for decision-making and defines the way interact with the markets. Our innovative research is aimed at the discovery of permanent value that persists in all market conditions. How does price form, what is volatility, and why will supply never balance demand – read about it in our research releases.
Our Partners
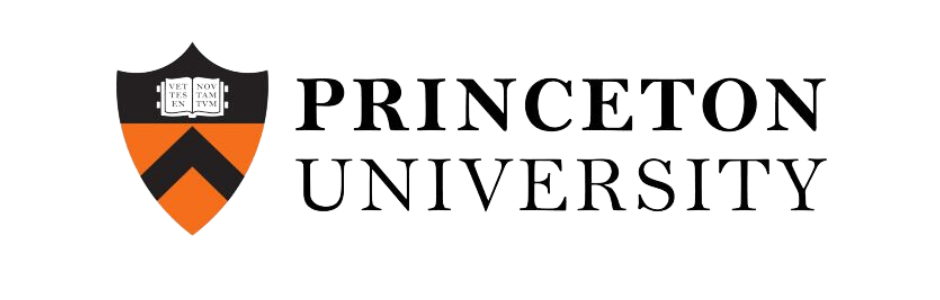
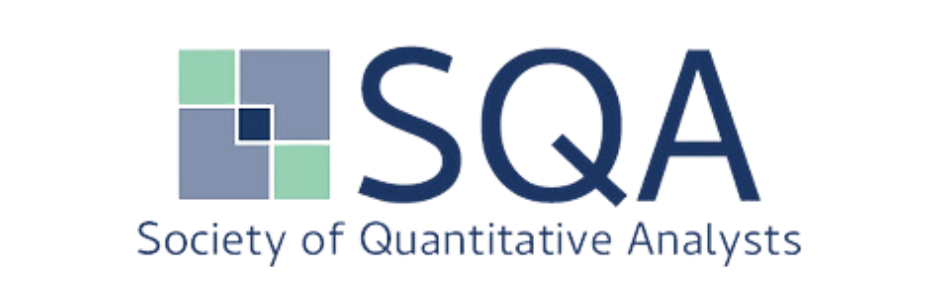
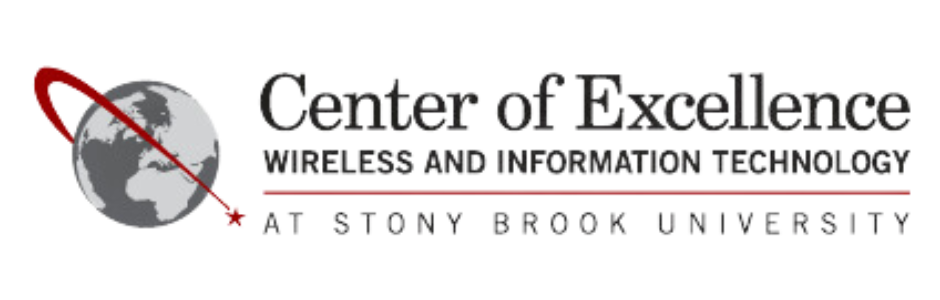
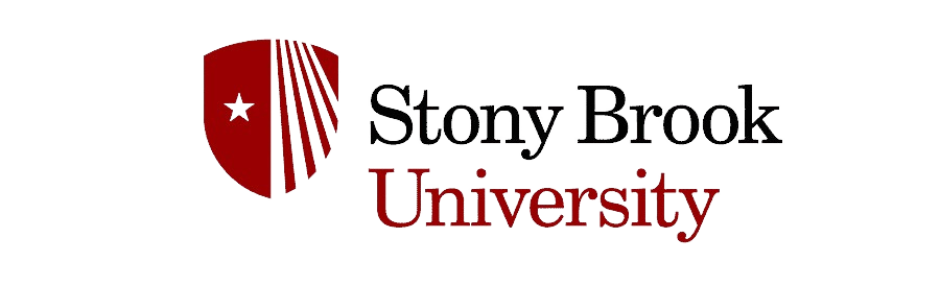
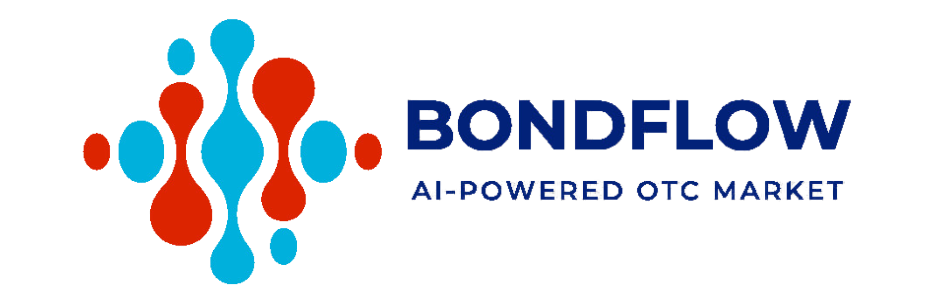
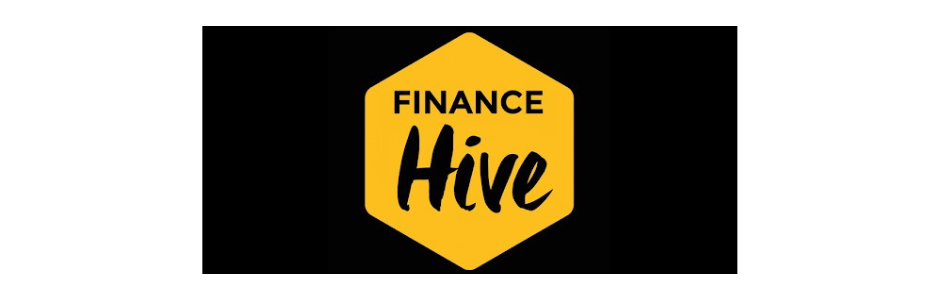
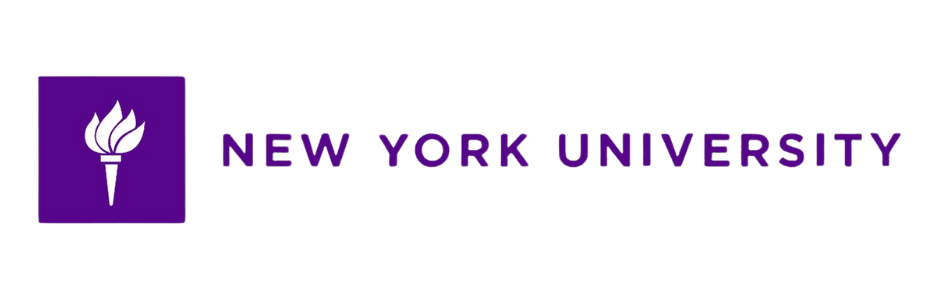
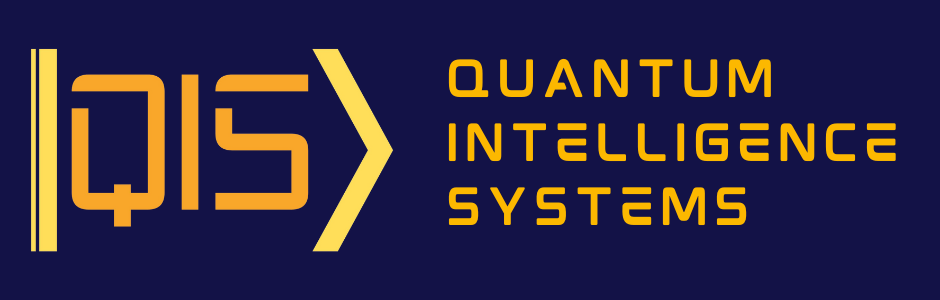
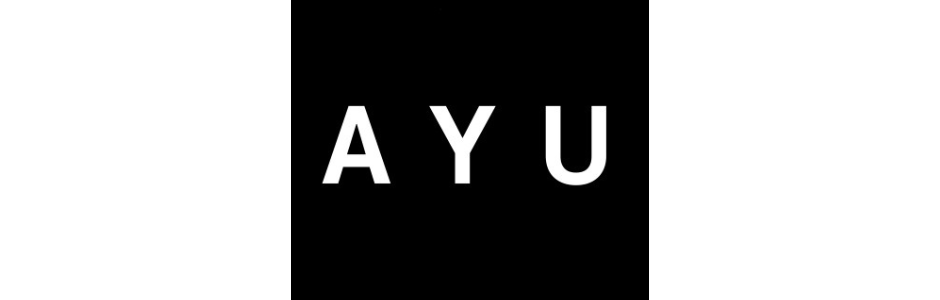
Board of Advisors
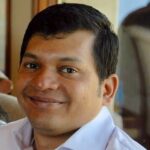
Shivkumar Yerradodi
Ex-Citadel Risk and Analytics Technology
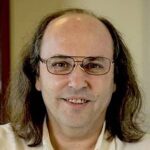
Raphael Douady
Notable Financial Mathematician
Professor
Centre d’Economie de la Sorbonne
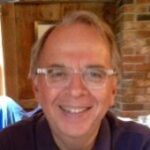
Bob Guzman
Chief Executive Officer Homology Digital Former Managing Director at BlackRock
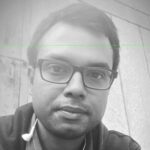
Pinaki Bhattacharyya
Risk Models and Standards
Wells Fargo, IISc
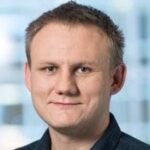
Pawel Polak
Stony Brook University, Professor of Applied Mathematics
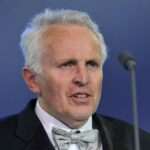
Alexander Polyakov
Fundamental Physics Prize Laureate, Princeton
Data, Decisions, Dollars: How Generative AI is Reshaping
Last week FMI Technologies was represented at the Quant Strats...
Read MoreFMI Technologies Sheds Light on Buy-Side Challenges Amidst
Jack Sarkissian, Managing Director at FMI Technologies, highlighted the “buzzsaw”...
Read MoreAI in Asset Management: Revolution or Evolution?
Delve into the cutting-edge intersection of quantum AI, financial markets,...
Read MoreContact Us
Asset Management
+1 212 220 7133
Research & Analytics
+1 212 220 7133
Liquidity Solutions
+1 212 729 8490
Press & Media
+1 212 220 7133